Amid an unprecedented global disruption, supply chains are grappling with unparalleled complexities and continue to face a multitude of challenges:
- Less than half (43%) forty-three percent of organizations have limited to no visibility of tier-one supplier performance. (KPMG)
- Interos Annual Global Supply Chain Report reveals that global supply chain disruptions cost large companies, on average, $182 million annually.
As businesses navigate these challenges, predictive analytics emerges as a beacon of clarity and precision, offering the ability to anticipate disruptions, optimize operations, and drive strategic decision making. By utilizing the power of data-driven insights, companies can transform their supply chain operations from reactive to proactive, ensuring resilience and agility in an increasingly unpredictable world. In this blog, we will delve into common challenges of the supply chain industry and how the implementation of predictive analytics enhances supply chain services.
Common challenges faced by the supply chain industry
1. Demand volatility and forecasting inaccuracy
Fluctuations in consumer demand lead to excess inventory or stockouts, both incur costs. Predicting these changes is increasingly difficult due to rapidly changing consumer preferences. Inaccurate demand forecasting makes it difficult to plan inventory levels, production schedules, and resource allocation.
2. Increase costs
Increasing costs for raw materials, transportation, labor, and compliance can erode profit margins. Supply chains must find ways to manage and optimize these costs without compromising service quality or delivery times.
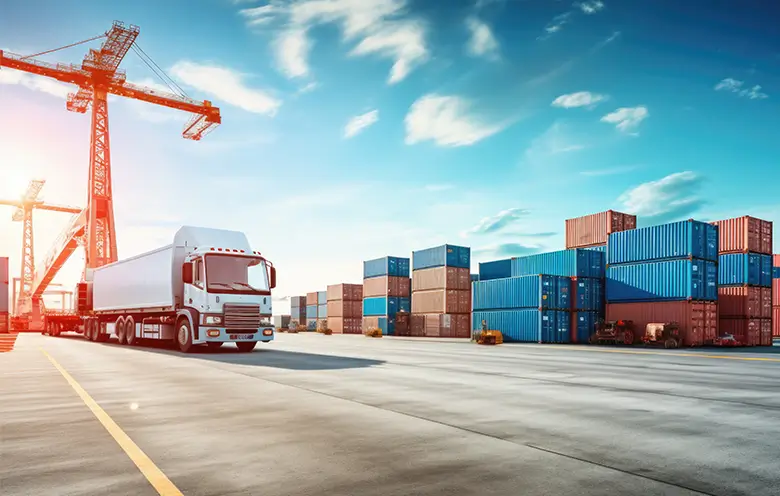
Smart logistics: Leveraging AI for superior supply chain management
AI revolutionizes logistic and supply chain management by automating repetitive tasks, enhancing productivity, and minimizing expenses. AI applications help businesses maintain a competitive edge.
3. Complexity in global supply chains
The globalization of supply chains has introduced a high level of complexity, involving multiple suppliers, manufacturers, and distributors across different countries. Managing these complex networks can be challenging, especially with varying regulations, tariffs, and trade agreements.
4. Visibility and transparency
Limited visibility into supply chain processes makes it difficult to monitor and track the movement of goods. Lack of transparency can lead to inefficiencies, increased risks of fraud, and challenges in maintaining quality control.
5. Sustainability
Increasing pressure from consumers and governments to adopt sustainable practices challenges supply chains to minimize their environmental footprint and often requires significant changes in sourcing, production, and logistics.
How predictive analytics enhances supply chain management
1. Improves demand forecasting
Predictive analytics helps businesses anticipate customer demands more accurately by analyzing historical sales data, market trends, and external factors like economic indicators and weather patterns. By improving demand forecasting accuracy, companies can reduce instances of stockouts and overstocking, ensuring optimal inventory levels and minimizing holding costs.
AI-driven forecasting can reduce supply chain errors by between 20 and 50 percent, according to McKinsey, leading to a 65 percent boost in efficiency through fewer lost sales and unavailable products.
For example, retailers can better predict which products will be in high demand during certain seasons or events, allowing for more strategic stocking decisions.
2. Enhances inventory management
With predictive analytics, companies can better understand inventory turnover rates and predict when to reorder stock. It minimizes excess inventory and storage costs ensuring products are available when needed. Predictive analytics optimizes inventory placement across multiple warehouses, reducing shipping times and costs.
For example, it is important for companies with global supply chains, where managing the flow of goods across borders and regions can be complex.
3. Proactive risk management
Predictive analytics enables companies to identify potential supply chain disruptions before they occur by analyzing data from various sources such as supplier reliability, geopolitical events, and natural disaster patterns. Businesses can assess risks and develop contingency plans allowing companies to mitigate risks, avoid costly delays, and maintain continuity in supply chain operations.
For example, if a key supplier is likely to face production issues due to political instability, companies can find alternative suppliers beforehand.
4. Optimizes transportation and logistics
Transportation is a significant cost driver in supply chain management. Predictive analytics can optimize transportation routes and schedules by analyzing traffic patterns, fuel prices, and delivery windows. It minimizes fuel consumption, reduces transportation costs, and ensures timely deliveries.
For example, predictive analytics helps in load optimization, ensuring that trucks are loaded as per their capacity, thereby reducing the number of trips required.
Suggested: How to prevent an 8% revenue loss through supply chain digitization?
5. Improves supplier relationship management
By analyzing supplier performance data, predictive analytics helps companies identify reliable suppliers and which one may pose risks. This insight allows companies to build stronger relationships with high-performing suppliers and address issues with underperforming ones. Predictive analytics helps to negotiate better terms and conditions with suppliers by providing data-driven insights into supplier performance and market trends.
Success stories of predictive analytics for supply chain sustainability
Walmart
According to a study by the University of Tennessee, the average cost of a supply chain disruption in the retail industry is $1.1 million.
Walmart, world’s largest retailer, has leveraged predictive analytics to enhance its demand forecasting and inventory management processes. By analyzing massive datasets, such as customer purchase history, seasonal trends, and local events, Walmart can accurately predict product demand at each store. This precision allows Walmart to optimize its inventory levels, reducing excess stock and minimizing the risk of stockouts. As a result, Walmart has significantly improved its supply chain efficiency, reduced waste, and enhanced customer satisfaction.
UPS
UPS, a global leader in logistics and package delivery, uses predictive analytics to optimize its delivery routes and improve fuel efficiency. By analyzing data on traffic patterns, weather conditions, and delivery locations, UPS can predict the most efficient routes for its delivery vehicles. This optimization enables to reduce fuel consumption, lower operational costs, and minimize environmental impact. It also provides more accurate delivery time estimates enhancing customer satisfaction.
DHL
According to a study by the Business Continuity Institute, the average cost of a supply chain disruption in the high-tech industry is $3.5 million.
DHL, a global leader in logistics and supply chain solutions, has successfully utilized predictive analytics to improve operational processes. By implementing predictive models, DHL has enhanced its ability to forecast demand, optimize delivery routes, and manage fleet maintenance.
For example, DHL uses predictive analytics to anticipate peak shipping times, allowing it to allocate resources more effectively and reduce delivery times. This proactive approach has improved customer satisfaction and cost efficiency. Additionally, predictive maintenance has enabled DHL to foresee and address potential vehicle breakdowns, minimizing downtime and ensuring timely deliveries.
Challenges in adopting predictive analytics and how to address them
1. Data quality and integration
Challenge
Predictive analytics in supply chain relies heavily on the quality and integration of data. Many organizations face challenges due to fragmented data sources, inconsistent formats, and incomplete or inaccurate data. Poor data quality can lead to unreliable predictions, undermining the effectiveness of analytics initiatives.
Solution
To address this challenge, companies must invest in robust data management practices. It includes implementing data governance frameworks that establish data quality standards, consistency, and accuracy. Integrating data from various sources into a unified system, using data lakes or warehouses, ensures predictive models access comprehensive and reliable data. Regular data audits and cleansing processes are critical to maintaining high data quality.
2. Lack of clear ROI measurement
Challenge
Measuring the return on investment (ROI) for predictive analytics projects can be difficult, particularly when benefits are indirect or long-term. Without clear metrics, it can be challenging to justify the investment in analytics initiatives.
Solution
Companies should define clear objectives and key performance indicators (KPIs) for predictive analytics projects. Regularly tracking and reporting on these KPIs can help demonstrate the value of analytics initiatives. Companies should also consider quantitative and qualitative benefits when assessing ROI, including cost savings, efficiency gains, and improved customer satisfaction.
3. Cost and resource constraints
Challenge
Implementing predictive analytics can be costly, and requires investment in technology, infrastructure, and talent. Small and medium-sized enterprises (SMEs) may face financial constraints that limit their ability to adopt advanced analytics solutions.
Solution
To manage costs, organizations can start with pilot projects focusing on specific areas of the supply chain where predictive analytics can deliver immediate benefits. This approach allows companies to test the waters and prove the value of analytics before scaling up. Cloud-based analytics solutions can also provide a cost-effective alternative, as they offer scalable resources without the need for significant upfront investment in infrastructure.
4. Data security and privacy concerns
Challenge
With the increased use of data, concerns about data security and privacy are growing. Companies must ensure that sensitive information is protected, and data usage complies with regulations such as GDPR and CCPA.
Solution
Organizations should implement strong data security measures, including encryption, access controls, and regular security audits. Establishing clear data privacy policies and ensuring compliance with relevant regulations are also crucial. Companies can build trust with customers and partners by demonstrating commitment to data security and privacy.
Optimize operational efficiency with efficient supply chain management
Predictive analytics in supply chain stands as a transformative force, empowering supply chains not only to survive but thrive in an increasingly complex and volatile global market. By utilizing the power of data to anticipate demand fluctuations, optimize inventory, and foresee potential disruptions, businesses can achieve unparalleled agility and resilience.
As predictive analytics continues to evolve, it enables organizations to turn uncertainty into a strategic advantage and drive sustained growth. Enhance your supply chain practices with Softweb Solutions by leveraging advanced data insights and expert guidance to stay ahead in a competitive market. Talk to our experts today and learn how we can implement predictive analytics to enhance your supply chain services.