Many companies, from large enterprises to mid-sized businesses, are having trouble accessing and utilizing the massive amounts of collected data. This means that they are not employing the power of data science. This term might seem a little daunting. But let me tell you, the power of data science is astounding. However, many organizations find it difficult to understand its importance. There are several myths that these businesses have regarding data science. Let’s bust them one-by-one.
Myth 1: Data science can only be applied on humongous data
IBM certainly has defined the 4 essential Vs of data science very well. These essential elements imply that you don’t necessarily need to have terabytes of data to perform data science upon. The other Vs apart from volume are: velocity, variety and veracity.
Velocity-based data: The size of the data isn’t the only thing that concerns data science, the velocity of the data generated also matters. Data generated in small amounts but at a wider pace is more likely to help you make the right decision at the right time.
Variety-based data: Data science can be applied to more varied data. The data can be from CRM systems, social media, and call logs. You can get better insights on your customer profiles, desires and so on, ultimately leading to better-informed decision making.
Veracity-based data: Historic data proves to be valuable. But if the data is more consolidated, conformed, and current, you are more likely to make the right decisions based on precise data set.
Hence, if you don’t have vintage experience in the business, data science can be applied to your data sets too. Data science applied to these data sets provides value to your business.
Myth 2: More data means higher accuracy
This is a myth every business looking for data science consulting should get rid of right away. More data may cause bigger potential oversights.
If you do not have a precise understanding of which data sets you need to analyze, you won’t know where to start, and it may actually be better to examine the issue on a smaller scale. This will help the data science model to understand a pattern and accordingly work on other data sets as well. Quantity does not always equal quality.
A small, yet well-maintained data set might have greater quality and monetize-able value, than a massive, but poorly governed database. If a sample set of the actual is representative and was used to develop a highly predictive correlation model, it might be possible to purge the majority of the big-data population without diminishing the business value.
More data doesn’t always imply good quality. Too many variables surge the complexity of the model without necessarily increasing accuracy or efficiency. More data always implies higher costs, more burdensome data collection and time-opportunity costs. Instead of choosing large data, opt for smart data. It is better to start analyzing internal datasets, before integrating them with public or external sources.
Myth 3: Data scientists will be replaced by artificial intelligence soon
Data scientist is termed as ‘the sexiest job of the century.
There is a fair chance that a machine (AI) will carry some data science activities in an autonomous
manner like data gathering and cleansing. However, a ‘data scientist’ will always be required to carry further operations and to tell the machine what needs to be done.
There is a drive to automate data science. People are building more sophisticated algorithms with a tendency to eliminate the need for a dedicated data scientist. However, that is highly unlikely to happen.
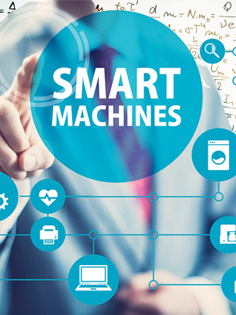
How AI and Data Science solve the biggest business challenges of today
Webinar agenda
- An Overview on Data Science and Artificial Intelligence
- Why every business needs Data Science and AI
- Business cases for varied industries
- Demo
- Roadmap for implementation
- Questions
Demand for data scientist’s skills is already sky high. And it will continue to increase in the estimative future. We will still need a person with sound judgment, and domain expertise to fetch important insights out of the processed data.
Myth 4: Learning a tool is the equivalent of learning data science
A data scientist isn’t just a programmer. Hence, knowing a tool or a mere programming language won’t make you a data scientist. Data science is beyond tools and algorithms. It requires skills and understanding of application of various predictive modelling techniques too.
Businesses looking for data scientists don’t just seek tool expertise. They seek someone who understands different aspects of data science like statistics, programming and business. However, learning a tool or a platform is equally essential. To learn data science an individual has to dive deeper into analytics and enhance the skills on various tools.
Myth 5: We can’t derive monetary benefits from data science
A 10% increase in data accessibility will result in more than $65 million additional net income for the typical Fortune 1000 company. – KPMG
To measure the financial or non-financial value of individual data items and of aggregated data sets, data science is necessary to be applied on to them.
Data science impacts the decisions related to customer acquisition, onboarding, retention, upsell, cross-sell and other concrete bottom-line indicators, as well as corresponding improvements in operational efficiency.
Applying data science on the data collected from your company’s marketing channels would enable you to understand which channels or sources are best for you. This will help you to make a decision on what medium you’d like to invest more in and would eliminate the resource that generates no value to your business. This indirectly will derive monetary benefit for your firm.
Myth 6: Data science is the same as business intelligence
People sometimes confuse business intelligence with data science.
Questions answered by business intelligence are:
• What
• When
• How
• Who
Business intelligence includes reporting, automated monitoring, interactive dashboards, scorecards, etc. It gathers the history of data, complies a report on it to give you a visualized presentation.
Data science, on the other hand, answers questions like;
• Why did it happen?
• Will it happen again?
• What will happen if we change the plan / product / service?
Data science includes data mining, statistical / quantitative analysis, predictive modeling, and multivariate testing. Data science helps you in forecasting and predicting while business intelligence empowers you with a visually appealing presentation of the current scenario.
Data science scenario now
If, being a business, your major concerns are how to outrun your competition and how to plan future products and services, data science will guide you through.
Data science helps businesses in many effective ways. The foremost step of applying data science is to come up with a methodological way to extract insightful information from data. Once the organization has the data requirement sorted, the next logical step would be to close the gap between data and decision-making, by viewing each business case as a data problem
If you have more such myths or misconceptions about data science, you may talk to our experts for further clarity on the subject.