Data is the backbone of modern analytics tools and machine learning algorithms, providing insights that enable leaders to drive impactful decisions and meet customer needs effectively. When utilized strategically, data becomes an asset for any organization.
463 exabytes of data will be generated each day by people as of 2025. – Raconteur
Today, organizations have access to abundant data and are poised to leverage its potential for comprehensive analytics. However, merely having access to data is not sufficient to overcome the numerous challenges faced in the digital transformation journey. Effective data management systems are essential, arising from the collaboration between IT and business teams.
Data drives every decision and solution, acting as a gateway to endless possibilities in today’s business environment. Data science has swiftly become a vibrant and promising field, drawing individuals eager to leverage its transformative potential. Yet, behind the charm of predictive analytics, machine learning, and artificial intelligence, there lies a critical foundation: data management. In this blog, we will explore the imperative role of data management in data science and its significance in ensuring the success and efficacy of data-driven initiatives.
Let’s begin with understanding what is data management
Data management is the comprehensive procedure of collecting, storing, organizing, and maintaining data to ensure its accuracy, accessibility, and reliability throughout its lifecycle. It encompasses a range of processes and tools designed to manage data as a valuable resource, enabling organizations to harness its full potential for data-driven decision making and strategic initiatives. Effective data management ensures that data is secure, consistent, and available when needed, forming the backbone of data science and analytics efforts.
The importance of data management in data science
Data management plays a foundational role that cannot be overstated in the data science domain. Effective data management is crucial for realising the full potential of data science initiatives, driving impactful business outcomes, and maintaining a competitive edge in a data-driven market. Data management underpins the success of data-driven initiatives through several essential functions:
- Data quality: It is important to have reliable and clean datasets that are crucial for generating trustworthy insights and models. Data management ensures the integrity and accuracy of data.
- Data accessibility: Data scientists must efficiently retrieve the information needed for their analysis. Data management facilitates easy and timely access to data.
- Data security and compliance: It is imperative to protect sensitive data and align data usage with regulatory requirements. Data management implements robust security measures and ensures compliance with legal and industry standards.
- Efficient data handling: It is crucial to streamline data organization and storage to facilitate efficient management of large datasets. Data management enhances data scientists’ ability to perform such complex analyses seamlessly.
- Scalability and collaboration: It is essential to support the scalability of data science projects and promote collaboration by providing a centralized, secure platform for data sharing and access. Efficient data management ensures effective project growth and team coordination.
By focusing on these aspects, data lifecycle management for data science boosts overall efficiency and impact, driving innovation and operational excellence.
Suggested: Why data analytics is important for your business?
Key elements of the data management process
Effective data management involves a series of strategic functions that ensure data is accurately processed, securely stored, and efficiently utilized. Key elements of this process include:
- Data architecture: Establishing a data architecture is fundamental, especially in large organizations. It involves creating a detailed framework for managing data, including documenting data assets and mapping data flows. This blueprint supports the deployment of databases and data platforms tailored to specific applications, ensuring structured data management.
- Database administration: Databases are central to storing and managing corporate data. This function encompasses designing, configuring, and maintaining databases used for both transaction processing and business intelligence. Key responsibilities include performance monitoring, tuning, data security, backup and recovery, and applying updates and patches to ensure optimal database performance and reliability. These elements are crucial for creating a robust data management strategy, enabling organizations to handle data effectively, support analytical processes, and drive business insights.
Types of data management
1. Data pipelines: Data pipelines automate workflows that streamline the flow of data from source to destination, enabling efficient data processing and analysis.
2. ETLs (Extract, Transform, Load): ETLs are built to extract data from various sources, transform it into a consistent format, and load it into a target system for analysis.
ETL vs ELT – understanding the key differences
Discover the key differences between ETL and ELT processes in data integration and how they can impact your business decisions. Read more to learn how to optimize your data pipeline for better performance and ROI.
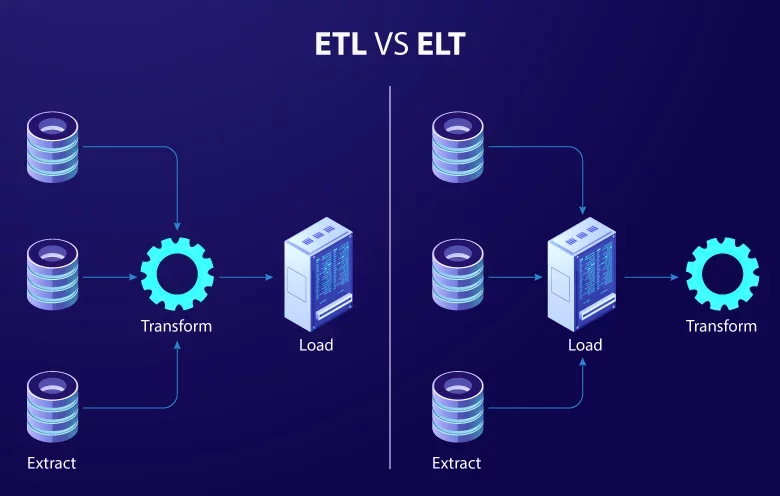
3. Data architecture: It provides the design and structure of data systems, such as databases and data models, to ensure efficient data management and scalability.
4. Data modeling: Data modeling defines how data is organized, stored, and related, ensuring efficient database design and supporting application development.
5. Data catalogs: They help document and organize data assets, making it easier to discover, understand, and manage data across the organization.
6. Data governance: It defines policies and procedures that ensure data quality, consistency, and security while ensuring compliance with regulatory requirements.
7. Data security: Data security ensures employing measures and controls to protect data from unauthorized access, breaches, and other security threats.
Other data management solutions:
- Data lifecycle management: This includes managing data from creation through disposal to ensure compliance and optimize storage.
- Data processing: Data processing transforms raw data through manipulation and analysis to extract valuable insights.
- Data integration: It combines data from different sources into a cohesive view for comprehensive analysis.
- Data migration: Data migration is the process of moving data between systems or storage solutions.
- Data storage: Data storage is the process of storing data efficiently, ensuring accessibility and durability.
The process of data management
Data delivery
It ensures that data and the insights derived from it are consistently and accurately distributed to both internal stakeholders and external customers, supporting informed decision-making and strategic initiatives.
Data governance
This involves establishing rigorous processes and best practices to maintain data availability, integrity, security, and usability, ensuring that data remains a reliable and secure asset within the organization.
Data operations (DataOps)
It adopts agile methodologies to design, deploy, and manage data applications across a distributed architecture. Similar to DevOps, this approach bridges the gap between development and IT operations, optimizing the entire data lifecycle.
By integrating these three elements, organizations can significantly enhance data quality, fortify data security, and improve the reliability of data-driven insights, leading to more strategic and effective data driven decision making.
Challenges of data management
Effective data management comes with several critical challenges that organizations must address to fully harness the power of their data. Key issues include:
- Data governance: Establishing comprehensive standards, policies, and procedures is crucial for maintaining data organization, preventing errors and duplication, and ensuring data integrity.
- Data quality: Implementing robust data quality checks and correction protocols is essential to address issues like errors, duplication, inconsistency, and incompleteness, ensuring accurate and reliable data.
- Data security: Adhering to data protection regulations such as GDPR and HIPAA requires strong security policies, access controls, and encryption strategies to protect data from unauthorized access and cyber threats.
- Data integration: Effectively combining data from various systems for analysis demands appropriate formatting, mapping, and transformation to ensure seamless integration and comprehensive insights.
- Data privacy: Safeguarding data against unauthorized access and complying with privacy laws involves implementing user restrictions, data encryption, and data retention policies to protect sensitive information.
Data management services help companies address these critical challenges in data science by ensuring data quality, accessibility, and security, which are essential for accurate analysis and informed decision-making.
Data management best practices
Adopting best practices in data management services is essential to mitigate challenges and enhance the value derived from data. Key practices include:
- Define strategic objectives: Establish clear business objectives to guide data management efforts. Focus on collecting and analyzing data that aligns with these goals to maximize relevance and efficiency. For example, if reducing customer churn is a goal, prioritize data that helps understand customer behavior and satisfaction.
- Craft robust data management strategies: Create documented procedures for data collection, organization, usage, and analysis. Collaborate with stakeholders to ensure these processes are efficient, compliant, and secure, providing a consistent framework for managing data.
- Guarantee data excellence: Prioritize the collection of accurate and relevant data to avoid the pitfalls of handling excessive, unhelpful information. Implement standards for data quality, such as mapping raw data to the correct fields and using verification tools to maintain accuracy.
Data management – The cornerstone of successful data science initiatives
By ensuring data quality, accessibility, security, and integration, organizations can realize the full potential of their data assets. Embracing best practices in data management not only mitigates challenges but also enhances the accuracy and reliability of insights, driving strategic decision-making and fostering innovation.
As businesses continue to navigate the complexities of the digital age, a robust data management strategy will remain pivotal in maintaining a competitive edge and achieving long-term success. With robust data management, data science services can deliver more precise and actionable insights, significantly impacting business growth. Connect with our expert data scientists to learn more.