One of the main objectives of inculcating the Industrial IoT is the automatic monitoring and detection of abnormal events, or changes and shifts in the collected data. However, most IIoT companies let over 50% of their data go unused. And this is a big challenge for businesses.
There are other challenges too, like:
- Latency issues that affect the results from real-time data
- The failure to predict when parts will breakdown
Why is anomaly detection critical for data-driven enterprises?
Today, companies have started to understand the importance of interconnected operations. Firms rely on real-time data to get a 360 degree view of their business. However, the voluminous data and the rush of coordinated activities taking place at scale make it challenging to spot anomalies.
Examples of potential anomalies:
- A leaking boiler resulting in the shutting down of the entire production line
- Too many failed login attempts signaling the possibility of fishy cyber activity
- Fraud detection in financial transactions
While the possibilities of anomaly detection are limitless, a single person or a traditional analytics system cannot study massive amounts of data to spot anomalies. There is a need for scalable systems that can automate the whole process.
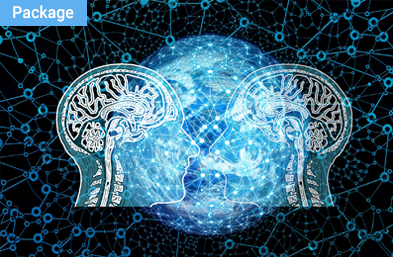
AI strategy and planning workshop
Artificial intelligence is transformational, and adopting this technology will enable companies to reshape their strategic vision so that they no longer rely on what they think is true, rather, leverage factual-based decision-making capabilities.
The solution: cognitive anomaly detection
Anomaly detection refers to the problem of finding patterns in data that don’t follow expected behavior. Datasets that don’t follow a pattern are termed as anomalies or outliers.
A cognitive approach to anomaly detection, powered by machine learning, AI, and advanced data analytics, provides businesses with solutions that help them to overcome the limitations of traditional statistical approaches. With cognitive predictive maintenance, all of the sensors can be measured in parallel.
When machine learning is enhanced with a cognitive IoT framework, it enables businesses to detect anomalies from the initial ingestion of sensor data. It also enables companies to quickly determine whether or not the abnormality in the pattern is an anomaly.
Detecting anomalies and increasing prediction accuracy
AI-powered algorithms help to create a self-learning system that learns from data patterns and delivers predictions or answers as required. AI for anomaly detection helps companies to improve the accuracy with which predictions are made.
Let’s see how the self-learning system works:
- The AI system is provided with datasets
- Data models are developed from the given datasets
- A potential anomaly is raised each time a transaction deviates from the model
- A domain expert approves the breach as an anomaly and acts upon the prediction
- The system learns from the action and builds upon the data model for future predictions
Automate dynamic problem detection
Identifying problems using the traditional reactive approach based on static thresholds doesn’t work for the current cloud infrastructure being used today. Normal behavior is constantly being redefined and the dynamic, ever-changing environments demand a new, proactive approach.
Social, mobility, IoT, wearables, AI personal assistants, and cloud are generating data in huge amounts which are intrinsically complex. It would be challenging to use traditional business intelligence systems to handle high volume and variety of data. Moreover, these traditional methods would require significant manual intervention for proper functioning.
Real-time anomaly detection, powered by machine learning and artificial intelligence, can help connect the dots between heterogeneous datasets. It helps businesses to see through the data and spot anomalies by comparing real-time data to ideal thresholds. Also, it helps companies to identify indicators and anomalies from various datasets to generate a pattern of how an ideal dataset would perform.
A company that continuously generates data from machines, systems, sensors, and applications must
leverage real-time anomaly detection. It gives end-to-end visibility of the business as well as helps narrow down on data instances that exhibit abnormal characteristics. It also helps them in taking proactive corrective actions that will save them from severe consequences like equipment downtime, equipment failure, financial mishaps, material losses, and more.
Prioritize problems automatically
Identify performance anomalies before they affect customers. Eliminate guesswork and stop spending time hunting down problems. AI algorithms autonomously help to determine whether a performance issue has an actual or potential impact on customers.
This gives businesses the means to gain control over enormous quantities of sensor data generating from every machine. This means augmented asset failure management, reduction of unplanned downtime, improved failure prediction, and enhanced asset life.
Softweb’s smart anomaly detection approach
With in-depth knowledge of data ecosystems, Softweb Solutions harnesses advanced analytics and continuous machine learning to auto-identify anomalies based on the metrics that best suits your requirements. Our AI-powered anomaly detection solution helps you find problems so you can focus on fixing them.
Contact our expert AI team to discuss more on the same.